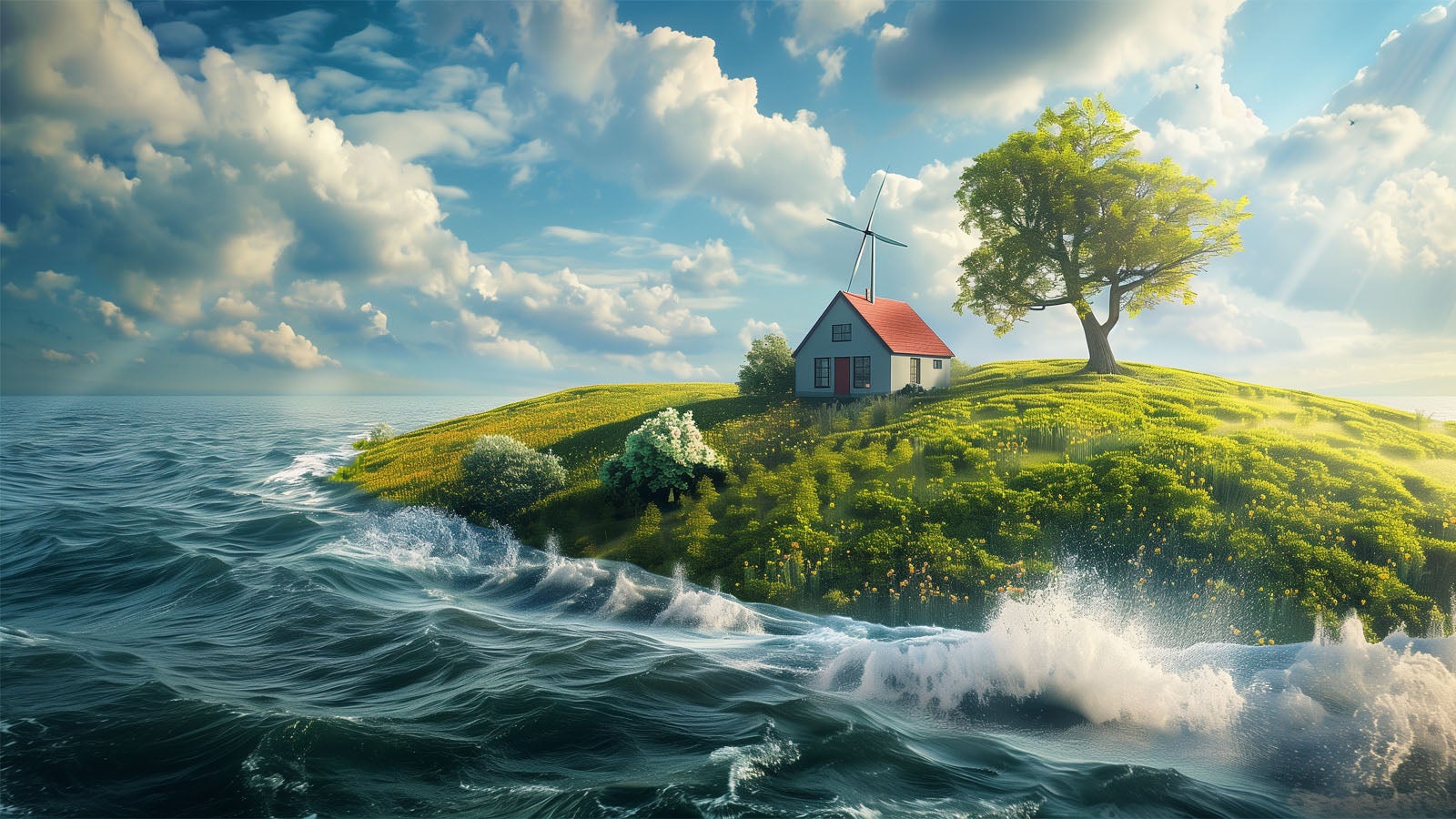
Encroaching sea levels are expected to intensify in the coming years, which, among other climate change factors, pose systemic risk to financial markets through physical damage to property, infrastructure and downstream environmental change.
Rice doctoral alumnus Zejian Liu and Meng Li, the Noah Harding Associate Professor of Statistics, have developed a unique plug-in process to model sea-level rates of change of multiple unknown functions.
“There are both measured and complex unknown factors to consider when analyzing sea-level trends across the world's regions,” said Liu. “Even though the effects of climate change, such as the melting of glaciers and ice sheets, have caused an increase in the total volume of ocean water, sea-level rise is not the same across the planet’s geographical features.”
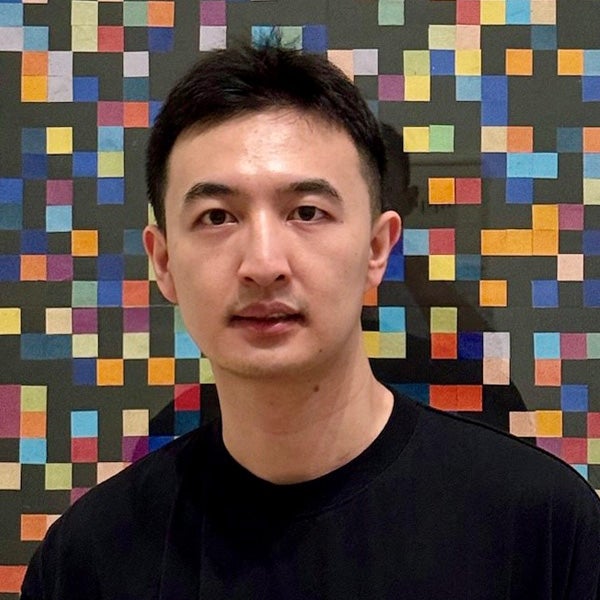
Liu, who completed his doctoral studies in statistics this past May with Li as his advisor, will present the paper Optimal Plug-In Gaussian Processes for Modeling Derivatives at the American Statistical Association’s Joint Statistical Meeting (JSM) in Portland, OR, this Aug 3-8.
Liu is one of four doctoral students from Rice’s Department of Statistics to present at JSM this year under a Rice Engineering Alumni (REA) Graduate Student Travel Grant. ASA’s Section on Bayesian Statistical Science (SBSS) also recognized Liu's research in January 2024.
“The problem of rising sea levels has been ongoing for several decades at a relatively slow rate. Subsequently, a lot of data has been collected from satellites, space missions, and networks of mobile ocean sensors,” explains Liu. “Bayesian models, such as Gaussian processes, are ideal for studying complex nonparametric patterns and serve as a building block in hierarchical models.”
Liu and Li’s algorithm is unique in the way it uses plug-in derivatives in the Gaussian process to analyze the rate of sea rise using global mean sea-level records in millimeters from coastal and island tide-gauge measurements from the late 19th to early 21st centuries.
“The optimal modeling strategy of derivatives and a data-driven hyperparameter allows us to tune and adapt the model for computational efficiency and rapid estimation,” explains Li.
Before joining Rice in 2017, Li was a visiting assistant professor in the Department of Statistical Science at Duke University. His research focuses on statistical modeling of challenging data that arise in scientific and industrial applications such as images, functional data, networks, and tree-structured data, with theoretical guarantees and scalable implementation.
Liu, who is now a principal data scientist at Capital One, says the inspiration behind his doctoral thesis was “the realization that traditional methods often fall short when capturing the nuances of complex and big data.”
More specifically, Liu’s thesis research focused on nonparametric statistical methods to study derivatives and localized characteristics of regression functions, utilizing techniques such as kernel methods and Gaussian processes. The work involved developing advanced statistical techniques for handling complex datasets that are theoretically robust and computationally efficient. Papers are currently under review for journal publication.
- Shawn Hutchins, Communications and Marketing Specialist