This spring, Rice University doctoral student Brian King has been counting more than just the days until graduation. The fifth-year graduate student has become an expert in developing Bayesian approaches to model count time-series, leading to better insight and improved forecasting.
Time-ordered, count-valued data is everywhere from financial markets to social networks to health care monitoring and often needs to be analyzed as it streams in.
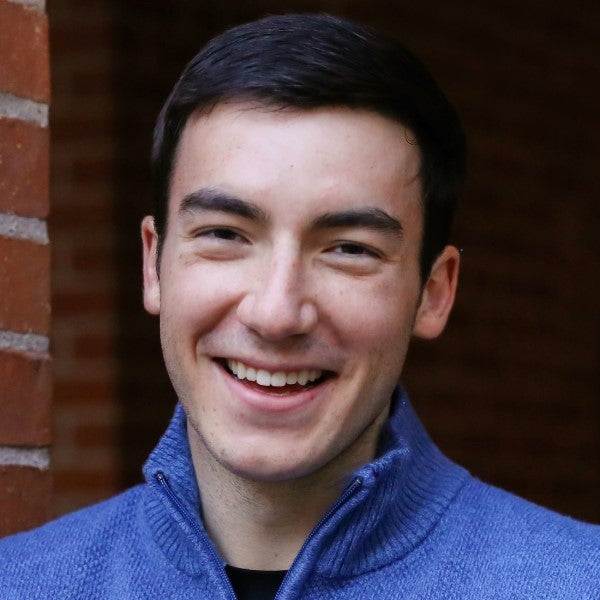
King’s doctoral research, which has been supported by a Graduate Research Fellowship from the National Science Foundation, has focused on distributional forecasting and uncertainty quantification for such time series.
“There is a pressing need for robust systems that can directly address the complexities of large-scale, time-dependent count data and give decision-makers the information they need in real-time, even when not all information is exactly known,” said King, who will begin working this July as a senior machine learning research engineer with Arm, a British semiconductor and software design company based in Cambridge, England.
Count data is poorly summarized by point estimates like a mean or even intervals. For optimal decision-making, predictions should come in the form of a full distribution, known as probabilistic forecasting. However, accurate probabilistic forecasts for count time-series are not easy to produce, since such data present a variety of complexities that make modeling difficult.
“For example, count data often inherit typical time series characteristics such as seasonality, but additionally exhibit numerous distributional features unique to the count setting, like zero-inflation, boundedness, over-or under dispersion and heaping,” added King, whose graduate research advisor is Dan Kowal, Rice’s Dobelman Family Assistant Professor in Statistics.
King is completing three research projects relating to modeling and forecasting of count time-series. Using CoFES cluster computing resources, the forecasting ability of these frameworks was demonstrated on simulated data as well as real-data applications.
In King’s first research project, he and Kowal introduced a broad class of multivariate state space models called the warped Dynamic Linear Model (warpDLM). The warpDLM connects count data to latent continuous data that can be modeled with a DLM through a warping operation composed of two parts: a rounding operator that ensures the correct support for the discrete data-generating process and a nonparametric transformation function that provides distributional flexibility.
King says, “The semiparametric framework we introduce adapts the powerful existing methods for time-series data in a way that allows for modeling the many complexities of count data. We develop conjugate inference for the warpDLM, enabling analytic and recursive updates, in turn facilitating the development of efficient algorithms for inference and forecasting.”
King’s second project took a different approach.
“Instead of training a model directly on a count time-series, we considered the scenario where several point forecasts for a count time-series are available and explored how these could be combined to output a calibrated probabilistic forecast,” said King.
To accomplish this task, he leveraged a flexible Bayesian count regression model that, akin to the warpDLM, performs Simultaneous Transformation and Rounding (STAR) of a latent continuous regression model. The resulting forecast combination approach (called STARcast) can produce calibrated and sharp distributional forecasts, even with only a small collection of point forecasts as the input.
King’s third project was of a more computational nature. He introduced a statistical software package, countSTAR, designed for both practitioners and researchers to use the count time-series methods introduced in his previous projects as well as a large variety of STAR models for static regression problems.
“In addition to including functionality for warpDLM modeling, countSTAR features unified syntax, useful output, and detailed online documentation. This package is now on CRAN, so we encourage any R users to install it and check it out!” said King.
- Shawn Hutchins, Communications and Marketing Specialist