The U.S. yield curve historically has been one of the most powerful predictors of future economic growth, inflation and recessions. As the coronavirus continues to affect global economies, economists are paying particular attention to the shape of the yield curve as an indicator of the country’s recovery.
Rice University data scientist Daniel Kowal, a specialist in statistical methods for large, complex and dependent datasets, built a dynamic regression model to analyze and forecast sequential bond yields across 40 years while incorporating key macroeconomic variables.
In a peer-review paper, published June 4 in an online advance publication in the journal Bayesian Analysis, Kowal says the Bayesian time series model, “Allows for the inclusion of information from past yield curves as well as the evolving effects of predictable or unpredictable events, such as unemployment and economic growth, shifts in financial policy, or COVID-19.”
Kowal, the Dobelman Family Assistant Professor of Statistics at Rice University, recently received a Young Investigator Award from the U.S. Army Research Office (ARO) for his development of optimal Bayesian approximations for targeted prediction.
“The great advantage of these regression models is that they can be used to more accurately capture important dynamic relationships between the forecast variable of interest, such as the yield curve, and scalar predictor variables that impact this curve,” Kowal said. “So if the economy is moving in certain directions, you have different features that can tell you how the yield curve is moving.”
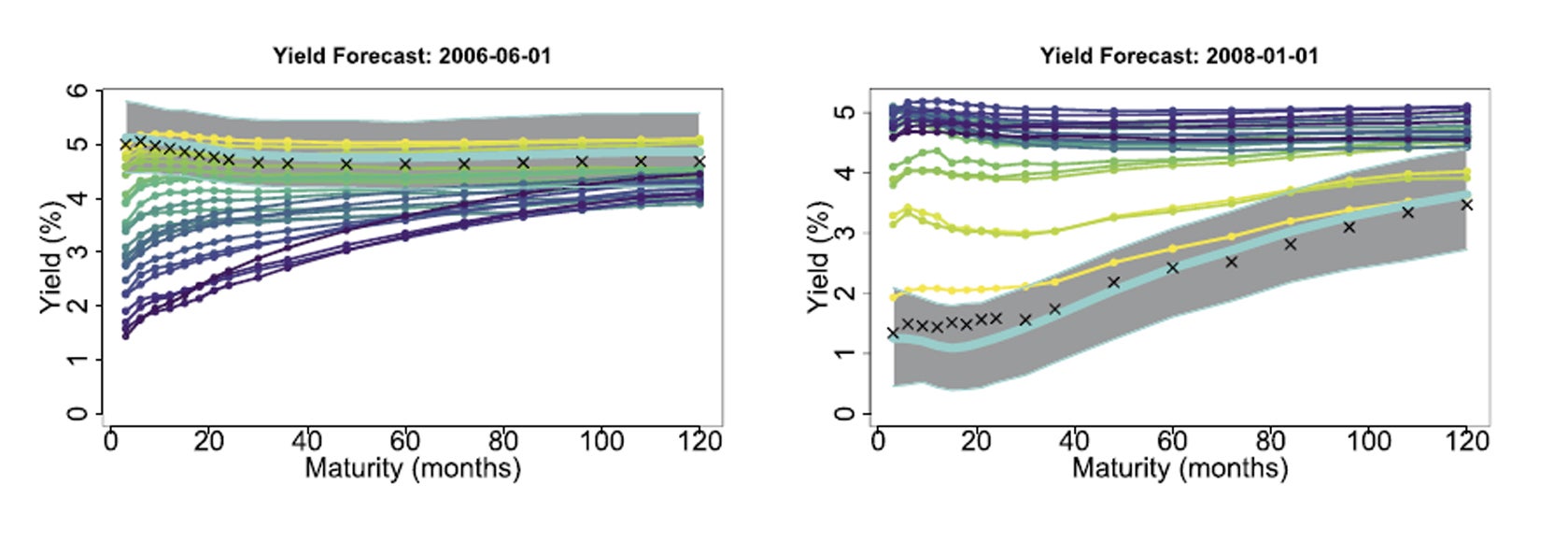
When Kowal and his research group build and fit complex models, they take special care to guard against overfitting. “Functional data present significant challenges for modeling and prediction as the data are usually highly-correlated, high-dimensional, and often unevenly spaced,” said Kowal. “Bayesian models are particularly useful tools in this setting: they can pull irrelevant predictors toward zero and encourage model simplicity in other ways.”
Recently, Kowal’s Bayesian models and algorithms have been applied for measles forecasting, physical activity monitoring, healthcare utilization, and, in ongoing research with Rice Statistics doctoral student Yunan Gao, intraday price curve modeling and forecasting.
- Shawn Hutchins, Communications and Marketing Specialist